Research of the Probabilistic Machine Learning Group
We develop new methods for probabilistic modeling, Bayesian inference and machine learning. Our current focuses are:
Agile Probabilistic AI
Collaborative decision making and design with AI
Multi-agent RL-based user modeling
Bayesian Deep Learning
Privacy-preserving and Fair AI
Simulator-based Inference
Applications
These topics match the research programs of
FCAI which we contribute to.
We develop and improve workflows for probabilistic modelling. Our core research is on designing computational diagnostics, devising methods and putting together AI tools that are interactive and can be generally applicable to iterative Bayesian model building workflows (see also https://fcai.fi/agile-probabilistic).
Our tools are modular, support explainability, and are developed independently of domain-specific applications. Our research lends itself to a wide range of modelling scenarios, such as Bayesian hierarchical models, decision making, causal models, Gaussian processes, time series analysis, and other probabilistic machine learning methods.
We actively contribute to popular probabilistic programming frameworks such as Stan and PyMC , and make our work widely available as part of free and modular open source software, including: projpred , loo , ArviZ , Bambi , bayesplot , priorsense , kulprit , cmdstanr and VAI-Lab .
Representative Publications
- Noa Kallioinen, Topi Paananen, Paul-Christian Bürkner, Aki Vehtari (2022). Detecting and diagnosing prior and likelihood sensitivity with power-scaling. [Preprint].
- Teemu Säilynoja, Paul-Christian Bürkner, Aki Vehtari (2022). Graphical Test for Discrete Uniformity and its Applications in Goodness of Fit Evaluation and Multiple Sample Comparison. Stat Comput 32. [Link].
- Akash Kumar Dhaka, Alejandro Catalina, Manushi Welandawe, Michael Riis Andersen, Jonathan Huggins, Aki Vehtari (2021). Challenges and Opportunities in High Dimensional Variational Inference. Advances in Neural Information Processing Systems 34 (NeurIPS 2021) [Link].
- Andrew Gelman, Aki Vehtari, Daniel Simpson, Charles C. Margossian, Bob Carpenter, Yuling Yao, Lauren Kennedy, Jonah Gabry, Paul-Christian Bürkner, Martin Modrák (2020). Bayesian workflow. [Preprint.]
- Andrew Gelman, Jennifer Hill, Aki Vehtari (2020). Regression and Other Stories (Analytical Methods for Social Research). Cambridge: Cambridge University Press. [Publisher's webpage for the book].
Most machine learning systems operate with us humans, to augment our skills and assist us in our tasks, such as decision-making, modeling, or design. In order for an AI agent to efficiently assist human users, it needs to learn a user model which takes into account their goals, plans, preferences and biases. These factors are latent and non-stationary, and it is challenging to infer them while requiring minimal effort from the user. To best elicit expert knowledge, we develop probabilistic strategies (e.g., active learning, Bayesian experimental design, Bayesian optimization) and associated inference techniques. This research contributes directly to the FCAI Interactive AI Virtual Laboratories.
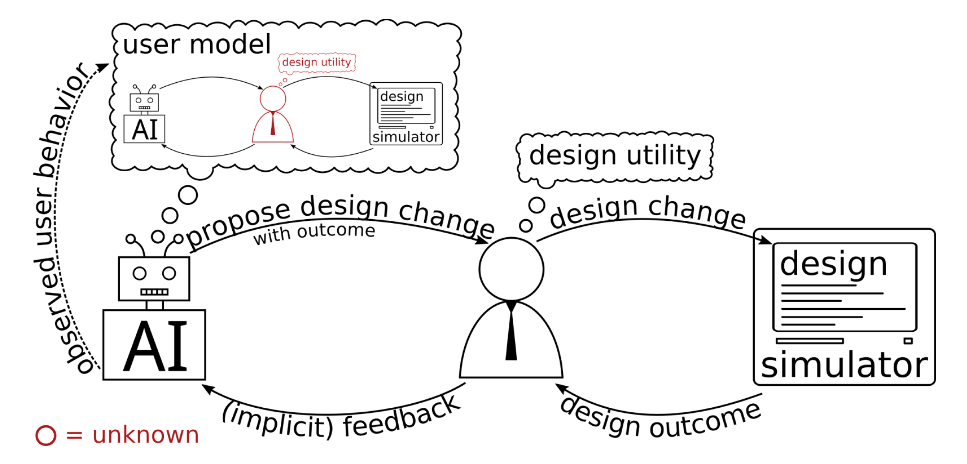
Representative Publications
- Mustafa Mert Çelikok, Pierre-Alexandre Murena, Samuel Kaski (2023). Modeling needs user modeling. Frontiers in Artificial Intelligence, 6:1097891. [Link].
- Iiris Sundin, Alexey Voronov, Haoping Xiao, Kostas Papadopoulos, Jannik Bjerrum, Markus Heinonen, Atanas Patronov, Samuel Kaski, Ola Engkvist (2022). Human-in-the-loop assisted de novo molecular design. [Preprint].
- Sebastiaan De Peuter, Antti Oulasvirta, Samuel Kaski (2023). Toward AI Assistants That Let Designers Design AI Magazine, 44(1):85-96. [Link].
- Sebastiaan De Peuter, Samuel Kaski (2023). Zero-shot assistance in sequential decision problems. Accepted for publication in Thirty-Seventh AAAI Conference on Artificial Intelligence (AAAI-23). [Link].
- Petrus Mikkola, Milica Todorovic, Jari Järvi, Patrick Rinke, Samuel Kaski (2020). Projective Preferential Bayesian Optimization. Proceedings of the 37th International Conference on Machine Learning, PMLR 119:6884-6892 (ICML 2020). [Link].
- Pedram Daee, Tomi Peltola, Aki Vehtari, Samuel Kaski (2018). IUI '18: 23rd International Conference on Intelligent User Interfaces Pages 305-310. [Link].
To create collaborative AI systems we need models of the users these systems will interact with. We develop user models that sufficiently capture human decision-making to be useful. Such models allow us to train AI agents that can understand and collaborate with human agents. These models are formulated using multi-agent reinforcement learning theory and learned from observed behaviour. The multi-agent formulation allows us to capture strategic behavior in humans (in which people actively try to influence another agent's inferences about themselves) by creating user models that model the user's beliefs about the AI system it is interacting with. Further, we base our user models on grounded cognitive theories, which allows us to minimize the amount of human interaction data needed to train them, and cope with biases and cognitive limitations that influence human behavior.
Our research further tackles methods needed to make these user models practically usable. A user model's parameters can be non-stationary yet they must be inferred from a limited amount of interaction data. We develop inference algorithms and surrogates based on amortization techniques that can help scale complex user models to practical application scenarios. See also https://github.com/AaltoPML/atom-team.
Representative Publications
- Tomi Peltola, Mustafa Mert Çelikok, Pedram Daee, Samuel Kaski (2019). Machine Teaching of Active Sequential Learners. Advances in Neural Information Processing Systems 32 (NeurIPS 2019). [Link].
- Fabio Colella, Pedram Daee, Jussi Jokinen, Antti Oulasvirta, Samuel Kaski (2020). UMAP '20: Proceedings of the 28th ACM Conference on User Modeling, Adaptation and Personalization. Pages 293-297. [Link].
- Antti Keurulainen, Isak Westerlund, Ariel Kwiatkowski, Samuel Kaski, Alexander Ilin (2021). Behaviour-Conditioned Policies for Cooperative Reinforcement Learning Tasks. ICANN 2021. Lecture Notes in Computer Science(), vol 12894. Springer, Cham. [Link].
- Mustafa Mert Çelikok, Pierre-Alexandre Murena, Samuel Kaski (2023). Accepted for publication in Thirty-Seventh AAAI Conference on Artificial Intelligence (AAAI-23). [Preprint].
- Alex Hämäläinen, Mustafa Mert Çelikok, Samuel Kaski (2023). Differentiable User Models. Accepted for publication in The 39th Conference on Uncertainty in Artificial Intelligence (UAI) 2023. Best paper award in NeurIPS HILL Workshop 2022 for a preliminary version. [Preliminary version].
Our goal is to develop principled Bayesian deep learning methods. These include:
- Develop novel priors and parameterisations of BDL for both large and small data.
- Analyse the manifold structures that emerge in such systems.
- Advance particle-based inference techniques for Bayesian sampling.
Representative Publications
- Tianyu Cui, Aki S. Havulinna, Pekka Marttinen, Samuel Kaski (2021). Informative Bayesian Neural Network Priors for Weak Signals. Bayesian Analysis. 17(4): 1121-1151. [Link].
- Trung Trinh, Markus Heinonen, Luigi Acerbi, Samuel Kaski (2022). Tackling covariate shift with node-based Bayesian neural networks. Proceedings of the 39th International Conference on Machine Learning (pp. 21751-21775). PMLR (ICML 2022). [Link].
- Simone Rossi, Markus Heinonen, Edwin Bonilla, Zheyang Shen, Maurizio Filippone (2021). Sparse Gaussian Processes Revisited: Bayesian Approaches to Inducing-Variable Approximations. 24th International Conference On Artificial Intelligence And Statistics (AISTATS), Proceedings of Machine Learning Research, Volume 130. [Link].
- Tianyu Cui, Yogesh Kumar, Pekka Marttinen, Samuel Kaski (2022). Deconfounded Representation Similarity for Comparison of Neural Networks. Advances in Neural Information Processing Systems 35 (NeurIPS 2022). [Link].
- Zheyang Shen, Markus Heinonen, Samuel Kaski (2021). De-randomizing MCMC dynamics with the diffusion Stein operator. Advances in Neural Information Processing Systems 34 (NeurIPS 2021). [Link].
Figure : In privacy-preserving learning, our aim is to learn important characteristics about the distribution of the data while protecting the anonymity of data subjects.
Machine learning models are capable of unintentionally capturing sensitive information underlying the training data, leading to disclosure of sensitive personal information - a privacy concern - as well as unfair decisions in automated processes - a fairness concern.
Privacy: We combine differential privacy, the prevailing formalism for anonymity, with Bayesian inference to develop powerful techniques for probabilistic modeling with rigorous privacy guarantees. We study the theoretical foundations and provide practical implementations for modern statistical inference under differential privacy, such as the software package Twinify, for creating and releasing anonymised synthetic twins of sensitive data sets.
Fairness: Since fairness is a contextual problem, we use a combination of probabilistic user modeling techniques to develop practical tools and intelligent agents which help human modelers develop fairer models within a given context.
Representative Publications
- Joonas Jälkö, Lukas Prediger, Antti Honkela, Samuel Kaski (2022). DPVIm: Differentially Private Variational Inference Improved. [Preprint].
- Lukas Prediger, Niki Loppi, Samuel Kaski, Antti Honkela (2022). d3p - A Python package for differentially-private probabilistic programming. Proceedings of Privacy Enhancing Technologies 2022(02). [Paper], [Code].
- Joonas Jälkö, Eemil Lagerspetz, Jari Haukka, Sasu Tarkoma, Antti Honkela, Samuel Kaski (2021). Privacy-preserving data sharing via probabilistic modelling. Patterns, 2:100271, 2021. [Link].
- Tejas Kulkarni, Joonas Jälkö, Samuel Kaski, Antti Koskela, Antti Honkela (2021). Differentially Private Bayesian Inference for Generalized Linear Models. Proceedings of the 38th International Conference on Machine Learning (pp. 5838-5849). PMLR (ICML 2021). [Paper].
Simulator-based inference (SBI) methods, or likelihood-free inference, such as approximate Bayesian computation (ABC) have gained popularity across scientific fields, as they enable statistical inference with complex, implicit models that only exist as computer simulators.
We focus on developing simulation-based inference methods that tackle challenging problems such as computationally costly, and misspecified simulators. For instance, we improve the robustness and sample efficiency of SBI methods by (a) eliciting knowledge from domain experts, and (b) exploiting structural and temporal information in the data. Additionally, we address these problems in multiple fields such as cognitive science, population genetics, neuroscience, radio propagation, and epidemiology, among others.
We develop the Engine for Likelihood-free Inference (ELFI), an open-source Python software library that is designed to be modular and extensible. It provides a wide range of simulator-based algorithms and convenient syntax for defining inference.
Representative Publications
- Ayush Bharti, Louis Filstroff, Samuel Kaski (2022). Approximate Bayesian Computation with Domain Expert in the Loop. In Proceedings of the 39th International Conference on Machine Learning (pp. 1893-1905) (ICML 2022). [Link].
- Alex Aushev, Henri Pesonen, Markus Heinonen, Jukka Corander, Samuel Kaski (2022). Likelihood-free inference with deep Gaussian processes. Computational Statistics and Data Analysis. [Link].
- Ayush Bharti, François-Xavier Briol, Troels Pedersen (2022). A General Method for Calibrating Stochastic Channel Models with Kernels. IEEE Transactions on Antennas and Propagation, , vol. 70, no. 6, pp. 3986-4001. [Link].
- Alexander Aushev, Anh Tran, Henri Pesonen, Andrew Howes, Samuel Kaski (2021). Likelihood-Free Inference in State-Space Models with Unknown Dynamics. [Preprint].
- Jarno Lintusaari, Henri Vuollekoski, Antti Kangasrääsiö, Kusti Skytén, Marko Järvenpää, Pekka Marttinen, Michael U. Gutmann, Aki Vehtari, Jukka Corander, Samuel Kaski (2018). ELFI: engine for likelihood-free inference. Journal of Machine Learning Research, 19(16):1\-7. [Link].
We work closely with researchers from other fields and industry partners to pursue breakthroughs in challenging real-world applications. Our contributions tackle central problems in healthcare, drug design, cognitive science, distributed computing, materials science, telecommunications, and robotics and autonomous systems. Aligned with the FCAI Virtual Labs initiative, our recent efforts mainly split into the following strands:
1. Health, genomics, and personalized medicine: We work with datasets from nationwide biobanks and electronic health records to support and improve the diagnosis and prevention of diseases. We are working with collaborators from the INTERVENE and FinRegistry projects.
2. Drug discovery: In collaboration with pharma companies, we aim to speed up and reduce failure rates in the drug discovery pipeline. We combine ML models with expert knowledge to improve molecule generation, molecular property prediction/optimization, and molecular dynamics simulation. We are also part of the EU-wide AIDD consortium.
3. Brain imaging: Our work on identifying features in MEG and EEG data with Bayesian methods helps to diagnose cognitive impairments such as dementia, which we investigate with our partners in the AI-Mind consortium.
Representative Publications
- Yogesh Verma, Samuel Kaski, Markus Heinonen, Vikas Garg (2022). Modular Flows: Differential Molecular Generation. Advances in Neural Information Processing Systems 35 (NeurIPS 2022). [Link].
- Tianyu Cui, Khaoula El Mekkaoui, Jaakko Reinvall, Aki S. Havulinna, Pekka Marttinen, Samuel Kaski (2022). Gene-gene interaction detection with deep learning. Communications Biology volume 5, Article number: 1238 (2022). [Link].
- Alexander Nikitin, Samuel Kaski (2022). KDD '22: Proceedings of the 28th ACM SIGKDD Conference on Knowledge Discovery and Data Minin Pages 3682-3690. [Link].
- Eero Siivola, Sebastian Weber, Aki Vehtari (2021). Qualifying drug dosing regimens in pediatrics using Gaussian processes. Statistics in Medicine. 2021; 40: 2355-2372. [Link].
- Eemeli Leppäaho, Hanna Renvall, Elina Salmela, Juha Kere, Riitta Salmelin, Samuel Kaski (2019). Discovering heritable modes of MEG spectral power. Human Brain Mapping, 40(5):1391-1402. [Link].